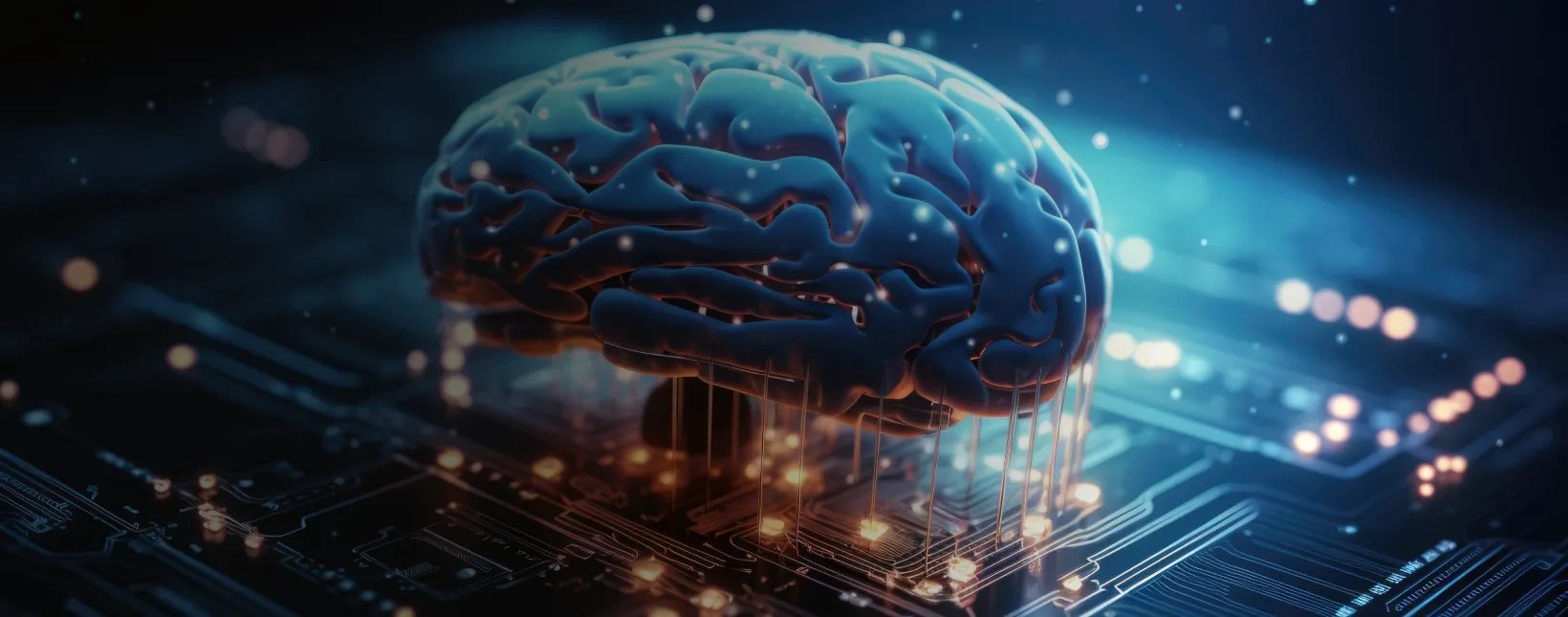
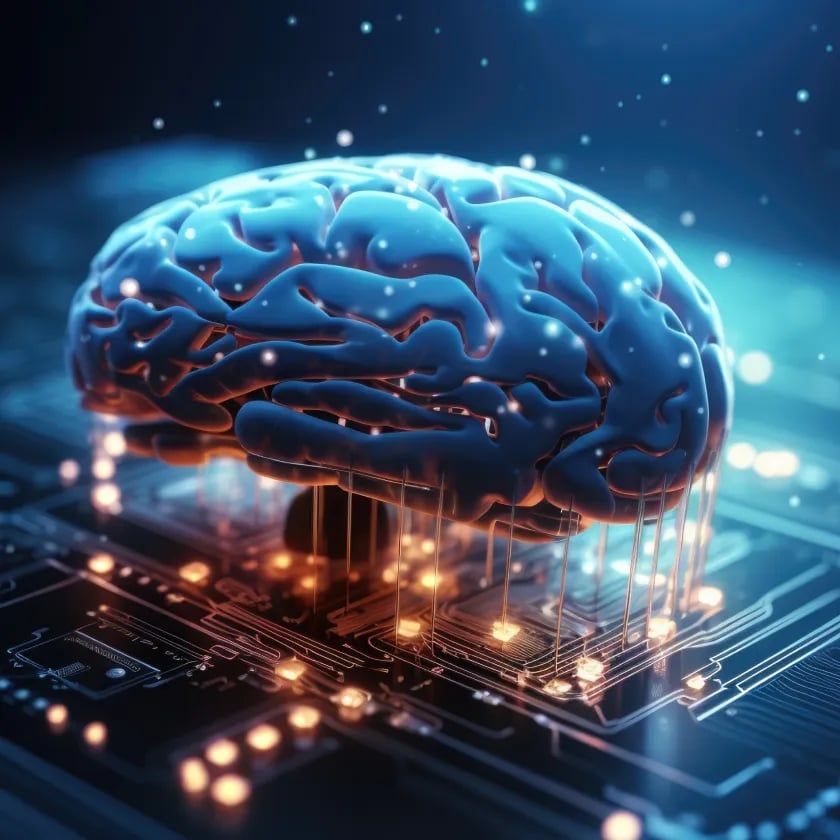
GN Quality Assurance
AI for the detection of defects
GN Quality Assurance is the Artificial Intelligence system created by GN Techonomy GN Techonomy for the identification, recognition and classification of production defects
GN Quality Assurance
Quality control has been a key factor in production ever since Henry Ford first introduced the assembly line. The idea was that if each individual had done their part in the production process optimally, the end product would have been of quality.
Nowadays, this belief has changed, also because the machines have replaced many manual processes. However, quality control remains one of the biggest problems: many companies bear costs related to quality which, due to production defects, reach up to 15-20% of the revenue. The European Commission has also estimated that in some sectors 50 of production is discarded, while in complex production lines this rate can even reach 90%.
For manufacturing companies, the biggest problem with regard to quality lies in the fact that a slight variation in the processes or materials used – often invisible to the human eye – can make the entire production defective. Therefore, to ensure that defective products do not reach the end user, companies have adopted extensive post-production quality control procedures. However, relying on manual post-product defect inspection practices implies that, for thousands of items, the defect is discovered only after incurring significant production costs.
.png)
In this context, modern technologies are inserted which offer companies multiple opportunities to improve routine processes that are no longer in step with the times. An application of Machine Learning techniques is linked to the analysis of images for the recognition and classification of objects. In this regard, GN Techonomy has created Artificial Intelligence systems specialized in identifying any manufacturing defects with greater advance in the production chain.
This approach combines advanced Computer Vision techniques, for analysis and pre-processing of images, with the use of Neural Networks for recognition and cataloging of defects: human intervention can thus be reduced by over 90%. In this way, the company will no longer be linked to the expert eye of the operator, but will have a quality monitoring system that will automatically analyze and catalog any errors and defective parts.
In addition, supervised self-learning techniques guide the evolution of the product over time, refining its precision. The flexibility of the approach makes this system adaptable and applicable to different types of product, ensuring widespread monitoring of production with consequent advantages in terms of costs, time and protection of product quality.
The Advantages of an Automated Optical Inspection are therefore manifold
- This system allows the timely recognition of true defects in the production processes, ensuring the high quality of the article before it passes from one production phase to another.
- It improves the process of recognizing real defects, with the possibility of identifying the exact defective piece and the possibility of replicating the model by extending it on multiple production lines.
- Significantly limits human intervention.
- It helps to collect historical and production statistics used to improve the entire production line.
- It helps to reduce material waste, repair and rework costs, as well as time and labor costs for production.
The Defect Identification Process consists in two phases
1. DEFECT IDENTIFICATION
In the first instance, sophisticated Computer Vision and Machine Vision techniques are used in order to identify, within the original image, a suspicious area that could be considered “anomalous”, cut out this detail and make a first classification based on the size error. Details are extracted for the images that meet the minimum requirements, in terms of size, so that they can be considered a possible defect. The images thus extracted are ready for the second step of the analysis process, namely the actual classification.
2. CLASSIFICATION
Secondly, a Convolutional Neural Network (CNN) is used for the classification of the cropped image as a defect or as a non-defect. The images cropped in the first step are given as input to the network which evaluates which of the two classes (defect / non defect), each image belongs to. CNN outputs a vector of two elements, each of which represents the probability of belonging to each of the two classes.
The images cataloged in defects / non defects are divided into three data sets:
- Data set - Training (60%)
- Data set - Validation (20%)
- Data set - Test (20%)
The training data set is used to train the neural network to distinguish between real and non-true defects. Subsequently, the validity of the model is tested, in terms of error and accuracy, by comparing the results obtained on the training and validation data sets. We proceed by modifying the hyper-parameters of the network until the desired results are achieved. In conclusion, the effectiveness of the model resulting from the test data set is verified.
GN Quality Assurance is therefore the starting point to get closer and closer to the “Golden Lot” and thus get to optimize your production process in a measurable way. This can then trigger product serving scenarios and new business models.
Main Reference:
- IRCE: Detection of manufacturing defects of Artificial Intelligence | 16/07/2020